How do you deal with Data Scientist Skill Shortage
- Subhas
- Feb 26, 2018
- 2 min read
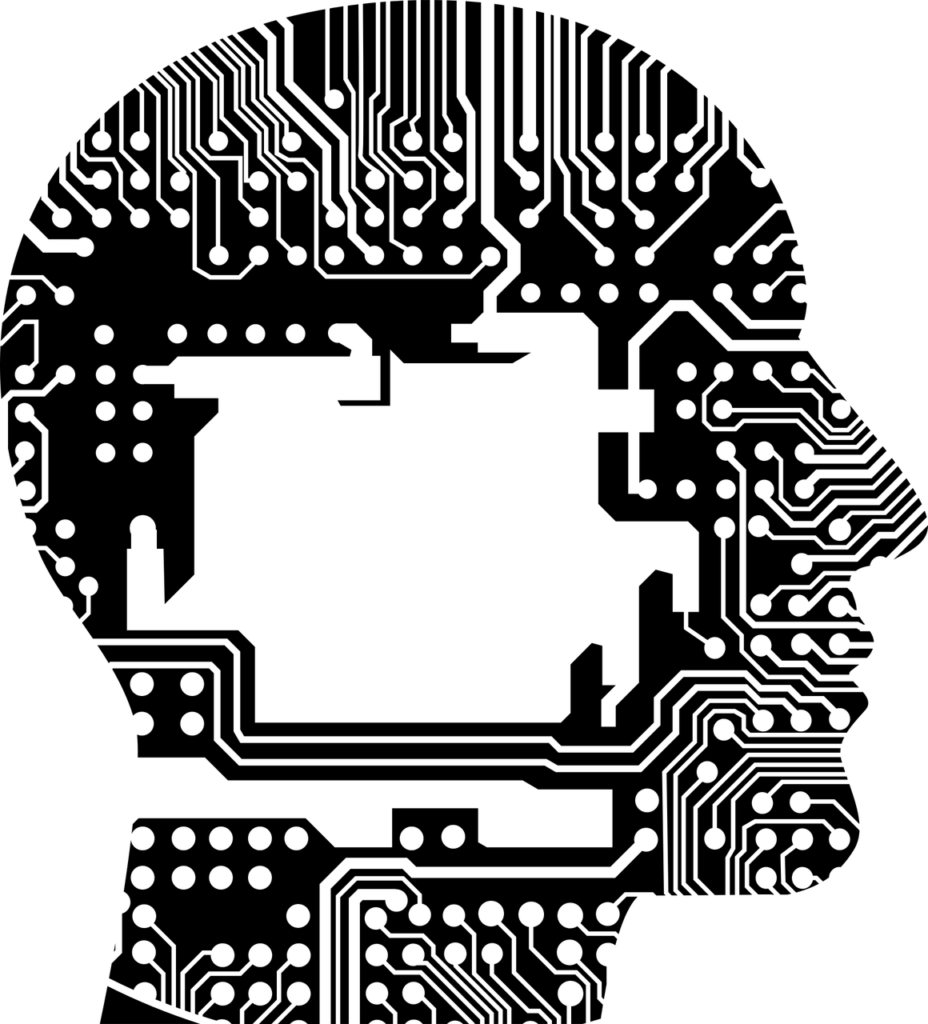
There will not be enough data scientists or AI specialists for every organization, and it may neither be economical nor necessary to have any in-house. The best way for digital technology to scale up is not through human labor. Instead, it is more efficient to have access to ready solutions, or a solid and specialized platform or tool covering data preparation, prediction, and visualization, to get more done with less time spent.
Whilst most organisations are still struggling to roll out digital programmes adding another team is a high risk strategy and costly.
We predict data scientist resources within the next 5 years are going to command a higher net worth then our talent folks in the city.
After all they are performing a very similar function through analysis and forecasting, armed with the right set of tools will unlock data silos and drive revenues.
We are already seeing DS roles in Silicon Valley commanding high end six figure salaries.
Based on the recent IDC Cognitive/Artificial Intelligence surveys, very positive sentiment has been reported among existing users of AI worldwide. In particular, 77% of organizations in the United States have found existing AI adoptions meeting or exceeding expectations; and 85% of organizations in Western Europe anticipate spending on AI to increase further in the next two years.
However, there is presently a higher demand for data scientists and AI specialists than the market can supply. 73% of organizations worldwide said they do not have data scientists or AI specialists in-house; and 57% of organizations cited the lack of data and skill sets as a primary challenge in the adoption of AI technology, more so than any other inhibitors.
Planning and deploying a team, integrating infrastructure and finding then right algorithms is just the start of it, it could take between 6-18 months to be operational.
Organisations should focus on solving real-world business problems pertinent to their core competency, and minimize attention on the technicality of the modeling process. Finding the right use cases and making agile use of the ever-increasing data assets so that everyone can become better at what they do, should be a matter of greater importance.
Investment decisions on AI technologies need to be carefully reviewed to consider factors including quality of result, time to value, data workers’ user experience, model transparency, and flexibility. An easy-to-use and outcome-guaranteed tool can hugely accelerate value delivery, and trigger a mindset change toward a data-driven and pro-innovation culture.
Many vendors are now offering AI technology platforms, which can be powered with little in-house expertise. Our forecast is smart organisation will lease this technology and the capability at least in the initial phases, until a clear business case can be put in place.
Comments